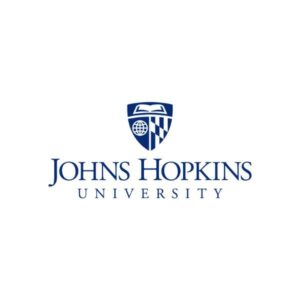
Johns Hopkins University, America’s first research university, has the Whiting School of Engineering and its mission to: Provide an outstanding engineering education that is innovative, rigorous, and relevant, and that prepares its graduates to be 21st century leaders; Advance the boundaries of engineering inquiry through research and the creation and dissemination of knowledge that improve the well-being of society; and Solve important societal problems through translational activities.
Department: Whiting School of Engineering
Homepage: https://engineering.jhu.edu/
E-mail: larry.nagahara@jhu.edu
We are a private sector organization and we plan to involve academic partners
- Looking for: Innovators/Entrepreneurs,Researcher (multidisciplinary),Researcher (scientific/technical/engineering)
- Track: Challenges,Open Ideas,Paired Teams
- Key application domains: Autonomous V2X,Energy Transition,Environment,Health,Industry 4.0,Lifelong Learning
Preferred hosting duration: No preference
Maximum number of fellows to be hosted by the organization simultaneously : 5
Challenge :
- AI for Design:
Our goal is to apply AI to the design of high-reliability, high-performance systems. We anticipate that AI will transform design at almost every level. Design tool providers are already integrating AI into their design software; for example, AutoDesk 360 has a generative design capability. What is less well developed, but arguably higher impact, is the application of AI to the system engineering process. System engineering provides the framework that connects the user needs to the engineering specifications, and which guides the process of going from a concept to a fully designed and tested product. Does generative AI provide a tool that can be used for the more human centric system engineering process? There are significant challenges including the fallible nature of generative AI. However, the ability for humans to interact with systems like ChatGPT in a more natural way, and the deep knowledge base generative AI draws on encourages exploration. After all, highly fallible humans produce highly reliable engineered systems. Could generative AI systems, help identify user needs and provide traceability of those needs to requirements? Or might generative AI provide a connective medium between design decisions made in nominally independent design teams? Could it identify and track dependencies which, for example, inform design tradeoff? We propose an experiment to test application of generative AI to an exemplar design problem (design of a robot) to understand how AI might be used most effectively in improving design processes. Success in this endeavor would open new avenues for the creation of complex systems, and have broad commercial impacts and societal benefits.
- AI for Design: