Italy
Researcher (scientific/technical/engineering)
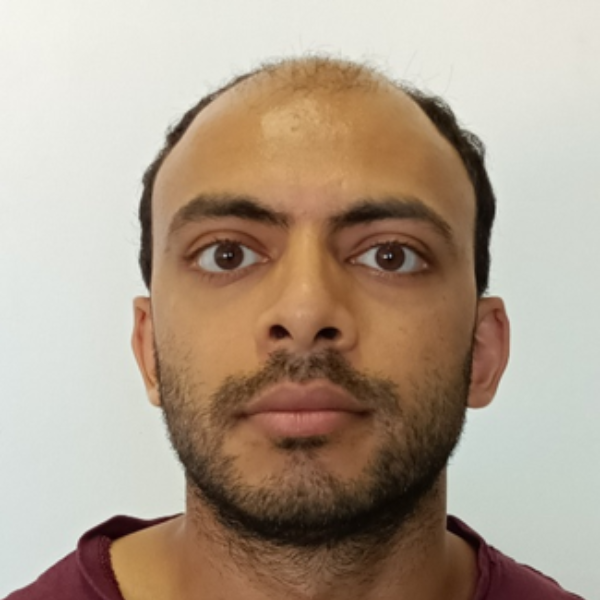
Date of the expedition
From 22/04/2024 to 31/07/2024
Selected Track
Paired Teams
Project title
Out of distribution generalization in quantum machine learning
Host Organization
University of Southern California
Media
Biography
I am a researcher in the field of quantum information, with particular interests in quantum metrology, quantum communications, and quantum machine learning.
I received my MSci in Theoretical Physics from University College London (First Class Honours) and then earned my PhD in Quantum Communications at the University of York.
I worked for two years as a postdoctoral research associate on the EU-funded QUARTET project before taking a position at the Istituto Nazionale di Fisica Nucleare (in Florence) as a postdoctoral researcher (assegno di ricerca). I have 15 published papers (orcid.org/0000-0002-0568-5737) that together have 2106 citations (according to Google Scholar).
Project Summary
Scientists increasingly use machine learning to learn about physical processes from experiments. In some fields, the outputs of those processes are quantum states. It is often difficult to obtain an exact mathematical description of how the parameters of an experiment map to the output. Instead, scientists may obtain sample data by carrying out the experiment multiple times and then apply machine learning to learn how the experiment acts “empirically”, i.e. from the data. Since the states involved are quantum, quantum machine learning promises to allow scientists to learn more from fewer experiments.
Any time we apply machine learning to a problem, an important question is how well our solution “generalizes”. We train our model on sample data, but we need to know whether it is still reliable when applied to unseen data. This problem is exacerbated when our sample data is drawn from a different distribution from the data that we want to carry out predictions on. This is called out of distribution generalization and is less studied and more challenging than in distribution generalization.
The goal of the project is to study out of distribution generalization in continuous variable (CV) systems (e.g., quantum optics). Whilst such settings could provide advantages over qubit-based quantum computing, they are even less studied. We intend to address this gap in the literature by providing a theoretical foundation for understanding how out of distribution generalization can work in CV systems.
Key Result
We have derived a result proving that out of distribution generalization is always possible for the task of learning CV quantum channels. This has wide applicability, not only in the field of theoretical quantum information, but also for a variety of related technologies and applications, such as metrology.
Impact of the Fellowship
Thanks to the fellowship, I was able to collaborate with Quntao Zhuang, an assistant professor at the University of Southern California, and his group. Quntao has significant expertise in the field of continuous variable quantum information. Together, we were able to develop an important result concerning out of distribution generalization that we will soon publish as a research paper in a high impact journal.
In addition, I was able to further research collaboration between USC and European research institutions, both directly – through the pending joint publication of a research paper – and indirectly, by building relationships with researchers in Quntao’s group that could lead to future collaboration.
The opportunity for this research to take place would not have existed without the fellowship.